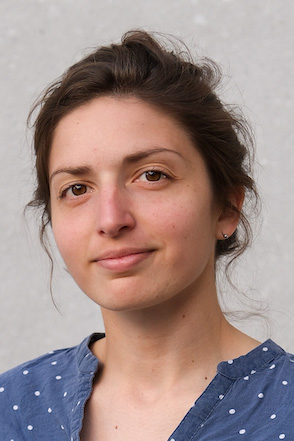
INSIGHT by Chiara Colesanti Senni (Council on Economic Policies) and Julia Anna Bingler (ETH Zurich)
| Climate risks are material financial risks for financial institutions. This fact is widely recognised by the academic literature and the financial community, from asset managers and investors, to central banks and financial regulators. As any other financial risk, climate risks must thus be accounted for and integrated into asset managers’ and investors’ decisions, as well as in central bank operations and financial supervision.
In order to quantify climate risks, appropriate metrics are needed. A widespread concern of asset managers, investors, central banks and supervisory authorities is that existing risk metrics do not provide sufficiently reliable assessments, due to the novelty of their methodologies and the lack of high-quality data. In this context, a problem might be that different climate risk metrics might deliver different results in terms of the exposure to climate risk of the same company.
On this account, a first remark is that some degree of divergence in the metrics produced by different providers is simply natural and does not represent an issue, given the high complexity and uncertainty characterizing climate risk assessments. Moreover, providers use different methodologies, assumptions and data inputs, which necessarily lead to some heterogeneity in the assessment. This diversity also captures different dimensions of exposure to climate risk. As a consequence, a general recommendation to users is to employ different metrics, so to obtain a more complete picture of the exposure to climate risk of their portfolio.
A second remark is that, despite their heterogeneity, metrics tend to converge for companies that are most and least exposed to climate risk. This is one of the findings from our recent study on whether climate risk exposure assessments at the company level differ, depending on the climate risk metrics adopted (Bingler et al. 2020). Asset managers and investors are hence advised to use climate risk information on these firms to better manage the risk profile of their portfolio. Central banks and supervisors could use such metrics to exclude high-risk assets from their balance sheets and identify risky investments undertaken by their supervised institutions.
| Our analysis
We undertake a preliminary analysis of the convergence of various transition risk metrics delivered by different providers.We base our analysis on theEuropean Central Bank’s (ECB) corporate bonds portfolio stemming from its Corporate Sector Purchase Program (CSPP). This portfolio gives us a sample of 287 firms, for which we obtained the assessments of exposure to transition risk from 10providers (Table 1), for a total of 12 metrics.
As each provider uses its own methodology, the risk metrics that they deliver are expressed in different units and scales. To homogenise the results, we rank, for each metric, the companies according to their exposure to transition risk. This way the different metrics become comparable among each other – although we loose information related to the magnitude of exposure to climate risk according to the different metrics.
Our analysis investigates the convergence of the rankings across different metrics, that is, their agreement on exposure to climate risk of the companies in our sample. From our pairwise correlation analysis, it appears that convergence in the assessment of firms’ risk exposures between metrics is in general relatively low. This result is illustrated in Figure 11, where the Spearman correlation coefficient, measuring the correlation in firms’ rank across different metrics, is depicted. Despite the generally low values obtained, more than half of the correlations computed are significantly different from zero (17 out of 28), which indicates that some degree of convergence between pairs of risk metrics exists. The relatively low correlation does not come as a surprise and it is not worrisome given the different approaches, modelling assumptions, data inputs and scenarios used by the different providers.
If we further disentangle our analysis according to the level of risk exposure of companies, an interesting pattern emerges: convergence is higher for companies that are most and least exposed to climate risk. This results is observable in Figure 2: Panel (a) displays the standard deviation of the assessments that we observe for each firm (y-axis), once firms are ranked according to their average rank across metrics (x-axis). The inverted U-shape indicates a lower standard deviation – and thus a higher convergence – in the case of most and least risk exposed companies. Panel (b) gives similar statistics, once the average ranks are aggregated by quintiles.2 The convergence of assessments for most and least climate risk exposed companies means that, despite the large heterogeneity existing in the approaches adopted by the different providers, they deliver a convergent picture on which companies mostly contribute to increase or decrease the risk exposure of a portfolio.
| Conclusion
The findings of our study show that while there exists a significant heterogeneity in the risk assessments delivered by different providers across our portfolio, risk metrics tend to converge on firms that are most and least exposed to transition risks. The overall relatively low convergence in assessments is not worrisome because of the complexity and uncertainty characterizing climate risk and because metrics very much differ in terms of the approach adopted and the assumptions made, as well as the data inputs used. This diversity contributes to a more comprehensive assessment of exposure to climate risk and allows to capture different dimensions of exposure to climate risk.
Our findings, although preliminary, allow us to draw recommendations for asset managers, investors, central banks and financial supervisors. Given the heterogeneity in risk assessments across risk metrics, we recommend users to rely on a set of metrics, rather than on a single one, to assess the exposure to transition risk of their portfolio or of their supervised institutions. Users should also always invest resources to carefully understand the methodology underlying the metrics adopted in order to identify the trade-offs implied by each methodology and choose the one that best fits their needs and beliefs.
We also recommend users to use a set of risk metrics to identify firms that are most and least exposed to transition risks, as risk metrics give a more convergent assessment for those firms than for firms which are moderately exposed to transition risks. Asset managers and investors can use this information to better manage the risk profile of their portfolio. Central banks and supervisors can use climate risk metrics to identify firms which are most exposed to transition risk, as these users are mostly concerned about not including high-risk assets in their balance sheets and about identifying risky investments undertaken by their supervised institutions, respectively. The available risk metricsare particularly useful to these goals as they tend to agree on the firms that are the most exposed to transition risk.
The lack of perfect data or perfectly coherent risk metrics cannot be used as a reason not to introduce climate risk metrics into asset managers and investors decisions, central bank operations and financial supervision. As the Governor of the Bank of England Andrew Bailey recently said: “uncertainty and lack of data is not an excuse”. When facing uncertainty and lack of data, asset managers and investors, as well as central banks and financial supervisors, should adopt a precautionary principle, according to which a higher risk than the one obtained with traditional risk metrics should be applied to assets exposed to climate risk. Moreover, heterogeneity in the assessment of exposure to climate risk is not necessarily an issue, as it depends on the different methodologies adopted by providers and reflects the fact that different providers are able to capture different dimensions of the exposure to climate risk.
1 Note that there are eight metrics, which are however not numbered one to eight because of the providers that we excluded from our core sample in which we excluded four metrics because they either do not cover a sufficient share of the portfolio, they only focus on emissions, they are not based on a forward-looking approach or on a firm-level analysis for all the firms.
2 The lower standard deviation on the extremes is partly by construction, as we consider a finite interval and average ranks. However, the outcome differs from the one that we would obtain assuming a uniform distribution, meaning that the standard deviation is indeed lower for most and least risk exposed companies. For more information, we refer the reader to Bingler et al. (2020).
〉Berg, Koelbel and Rigobon (2019). “Aggregate confusion: the divergence of ESG ratings”, MIT Sloan School Working Paper 5822-19.
〉Bingler, Colesanti Senni (2020). “Taming the Green Swan: How to improve climate-related financial risk assessments”, CER-ETH Economics Working Paper Series.
〉Bingler, Colesanti Senni, Monnin (2020). “Climate Financial Risks: Assessing Convergence, Exploring Diversity”, Council on Economic Policies Discussion Note.
〉Monnin (2018). “Integrating Climate Risks into Credit Risk Assessment-Current Methodologies and the Case of Central Banks Corporate Bond Purchases”, Council on Economic Policies Discussion Note.
〉Semieniuk, Campiglio,Mercure, Volz and Edwards (2019). “Low-carbon transition risks for finance”, SOAS Department of Economics Working Paper.
| brief bio
Chiara Colesanti Senni is a fellow with the Council on Economic Policies in Zurich (CEP) where she focuses on the integration of environmental risks in monetary policy. Prior to that she has been doing her PhD in Resource Economics at ETH Zurich. During this time she focused on the transition to a low-carbon economy, including the possibility for central banks to adopt emission-based interest rates in their lending facilities.
Julia Anna Bingler is a Doctoral Researcher at the Center of Economic Research at ETH Zurich. Her research focuses on climate transition risk analyses and TCFD-related disclosures. Prior to joining ETH, she worked for various academic institutions and think tanks on sustainable finance regulatory approaches and market-based climate policy. Besides her research, Julia Anna Bingler monitors national and international climate policy processes and participated in various UNFCCC climate conferences as advisor and observer on finance-related topics.
| about
ETH Zurich in Switzerland has been founded in 1855 as Swiss center of innovation and knowledge in science and technology. It regularly ranks as one of the world’s top universities in international league tables. The Center of Economic Research at ETH Zurich (CER-ETH) conducts high-quality scientific research in economics. It specializes in public economics, energy and resource economics, macroeconomics, international economics, innovation and risk analysis, and other fields of micro- and macroeconomics.
The Council on Economic Policies (CEP) is a non-profit economic policy think tank for sustainability, focused on fiscal, monetary and trade policy. Based in Zurich, Switzerland, CEP’s goal is to leverage existing analysis, fill research gaps and translate findings into policy recommendations on a national and international scale. CEP also collaborates closely with third parties and supports the launch of new organizations to build alliances and increase impact.
| All opinions expressed are those of the author. investESG.eu is an independent and neutral platform dedicated to generating debate around ESG investing topics.